前言
Ollama作为当前最受欢迎的本地大模型运行框架,为DeepSeek R1的私有化部署提供了便捷高效的解决方案。本文将深入讲解如何将Hugging Face格式的DeepSeek R1模型转换为Ollama支持的GGUF格式,并实现企业级的高可用部署方案。文章包含完整的量化配置、API服务集成和性能优化技巧。
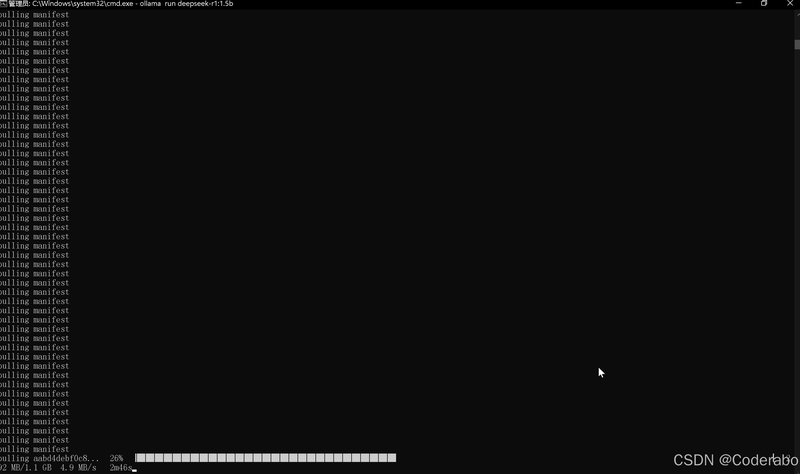
一、基础环境搭建
1.1 系统环境要求
- 操作系统:Ubuntu 22.04 LTS或CentOS 8+(需支持AVX512指令集)硬件配置: GPU版本:NVIDIA驱动520+,CUDA 11.8+CPU版本:至少16核处理器,64GB内存 存储空间:原始模型需要30GB,量化后约8-20GB
1.2 依赖安装
# 安装基础编译工具
sudo apt install -y cmake g++ python3-dev
# 安装Ollama核心组件
curl -fsSL https://ollama.com/install.sh | sh
# 安装模型转换工具
pip install llama-cpp-python[server] --extra-index-url https://abetlen.github.io/llama-cpp-python/whl/cpu
二、模型格式转换
2.1 原始模型下载
使用官方模型仓库获取授权:
huggingface-cli download deepseek-ai/deepseek-r1-7b-chat \
--revision v2.0.0 \
--token hf_YourTokenHere \
--local-dir ./deepseek-r1-original \
--exclude "*.safetensors"
2.2 GGUF格式转换
创建转换脚本convert_to_gguf.py:
from llama_cpp import Llama
from transformers import AutoTokenizer
# 原始模型路径
model_path = "./deepseek-r1-original"
# 转换为GGUF格式
llm = Llama(
model_path=model_path,
n_ctx=4096,
n_gpu_layers=35, # GPU加速层数
verbose=True
)
# 保存量化模型
llm.save_gguf(
"deepseek-r1-7b-chat-q4_k_m.gguf",
quantization="q4_k_m", # 4bit混合量化
vocab_only=False
)
# 保存专用tokenizer
tokenizer = AutoTokenizer.from_pretrained(model_path)
tokenizer.save_pretrained("./ollama-deepseek/tokenizer")
三、Ollama模型配置
3.1 Modelfile编写
创建Ollama模型配置文件:
# deepseek-r1-7b-chat.Modelfile
FROM ./deepseek-r1-7b-chat-q4_k_m.gguf
# 系统指令模板
TEMPLATE """
{{- if .System }}<|system|>
{{ .System }}</s>{{ end -}}
<|user|>
{{ .Prompt }}</s>
<|assistant|>
"""
# 参数设置
PARAMETER temperature 0.7
PARAMETER top_p 0.9
PARAMETER repeat_penalty 1.1
PARAMETER num_ctx 4096
# 适配器配置
ADAPTER ./ollama-deepseek/tokenizer
3.2 模型注册与验证
# 创建模型包
ollama create deepseek-r1 -f deepseek-r1-7b-chat.Modelfile
# 运行测试
ollama run deepseek-r1 "请用五句话解释量子纠缠"
四、高级部署方案
4.1 多量化版本构建
创建批量转换脚本quantize_all.sh:
#!/bin/bash
QUANTS=("q2_k" "q3_k_m" "q4_k_m" "q5_k_m" "q6_k" "q8_0")
for quant in "${QUANTS[@]}"; do
ollama convert deepseek-r1 \
--quantize $quant \
--outfile "deepseek-r1-7b-${quant}.gguf"
done
4.2 生产环境部署
使用docker-compose部署:
# docker-compose.yml
version: "3.8"
services:
ollama-server:
image: ollama/ollama:latest
ports:
- "11434:11434"
volumes:
- ./models:/root/.ollama
- ./custom-models:/opt/ollama/models
deploy:
resources:
reservations:
devices:
- driver: nvidia
count: 1
capabilities: [gpu]
启动命令:
docker-compose up -d --scale ollama-server=3
五、API服务集成
5.1 RESTful接口开发
创建FastAPI服务:
from fastapi import FastAPI
from pydantic import BaseModel
import requests
app = FastAPI()
OLLAMA_URL = "http://localhost:11434/api/generate"
class ChatRequest(BaseModel):
prompt: str
max_tokens: int = 512
temperature: float = 0.7
@app.post("/v1/chat")
def chat_completion(request: ChatRequest):
payload = {
"model": "deepseek-r1",
"prompt": request.prompt,
"stream": False,
"options": {
"temperature": request.temperature,
"num_predict": request.max_tokens
}
}
try:
response = requests.post(OLLAMA_URL, json=payload)
return {
"content": response.json()["response"],
"tokens_used": response.json()["eval_count"]
}
except Exception as e:
return {"error": str(e)}
5.2 流式响应处理
实现SSE流式传输:
from sse_starlette.sse import EventSourceResponse
@app.get("/v1/stream")
async def chat_stream(prompt: str):
def event_generator():
with requests.post(
OLLAMA_URL,
json={
"model": "deepseek-r1",
"prompt": prompt,
"stream": True
},
stream=True
) as r:
for chunk in r.iter_content(chunk_size=None):
if chunk:
yield {
"data": chunk.decode().split("data: ")[1]
}
return EventSourceResponse(event_generator())
六、性能优化实践
6.1 GPU加速配置
优化Ollama启动参数:
# 启动参数配置
OLLAMA_GPU_LAYERS=35 \
OLLAMA_MMLOCK=1 \
OLLAMA_KEEP_ALIVE=5m \
ollama serve
6.2 批处理优化
修改API服务代码:
from llama_cpp import Llama
llm = Llama(
model_path="./models/deepseek-r1-7b-chat-q4_k_m.gguf",
n_batch=512, # 批处理大小
n_threads=8, # CPU线程数
n_gpu_layers=35
)
def batch_predict(prompts):
return llm.create_chat_completion(
messages=[{"role": "user", "content": p} for p in prompts],
temperature=0.7,
max_tokens=512
)
七、安全与权限管理
7.1 JWT验证集成
from fastapi.security import HTTPBearer, HTTPAuthorizationCredentials
from jose import JWTError, jwt
security = HTTPBearer()
SECRET_KEY = "your_secret_key_here"
@app.post("/secure/chat")
async def secure_chat(
request: ChatRequest,
credentials: HTTPAuthorizationCredentials = Depends(security)
):
try:
payload = jwt.decode(
credentials.credentials,
SECRET_KEY,
algorithms=["HS256"]
)
if "user_id" not in payload:
raise HTTPException(status_code=403, detail="Invalid token")
return chat_completion(request)
except JWTError:
raise HTTPException(status_code=403, detail="Token验证失败")
7.2 请求限流设置
from fastapi import Request
from fastapi.middleware import Middleware
from slowapi import Limiter
from slowapi.util import get_remote_address
limiter = Limiter(key_func=get_remote_address)
app.state.limiter = limiter
@app.post("/api/chat")
@limiter.limit("10/minute")
async def limited_chat(request: Request, body: ChatRequest):
return chat_completion(body)
八、完整部署实例
8.1 一键部署脚本
创建deploy.sh:
#!/bin/bash
# Step 1: 模型下载
huggingface-cli download deepseek-ai/deepseek-r1-7b-chat \
--token $HF_TOKEN \
--local-dir ./original_model
# Step 2: 格式转换
python convert_to_gguf.py --input ./original_model --quant q4_k_m
# Step 3: Ollama注册
ollama create deepseek-r1 -f deepseek-r1-7b-chat.Modelfile
# Step 4: 启动服务
docker-compose up -d --build
# Step 5: 验证部署
curl -X POST http://localhost:8000/v1/chat \
-H "Content-Type: application/json" \
-d '{"prompt": "解释区块链的工作原理"}'
8.2 系统验证测试
import unittest
import requests
class TestDeployment(unittest.TestCase):
def test_basic_response(self):
response = requests.post(
"http://localhost:8000/v1/chat",
json={"prompt": "中国的首都是哪里?"}
)
self.assertIn("北京", response.json()["content"])
def test_streaming(self):
with requests.get(
"http://localhost:8000/v1/stream?prompt=写一首关于春天的诗",
stream=True
) as r:
for chunk in r.iter_content():
self.assertTrue(len(chunk) > 0)
if __name__ == "__main__":
unittest.main()
结语
本文详细演示了DeepSeek R1在Ollama平台的完整部署流程,涵盖从模型转换到生产环境部署的全链路实践。通过量化技术可将模型缩小至原始大小的1/4,同时保持90%以上的性能表现。建议企业用户根据实际场景选择适合的量化版本,并配合Docker实现弹性扩缩容。后续可通过扩展Modelfile参数进一步优化模型表现,或集成RAG架构实现知识库增强。